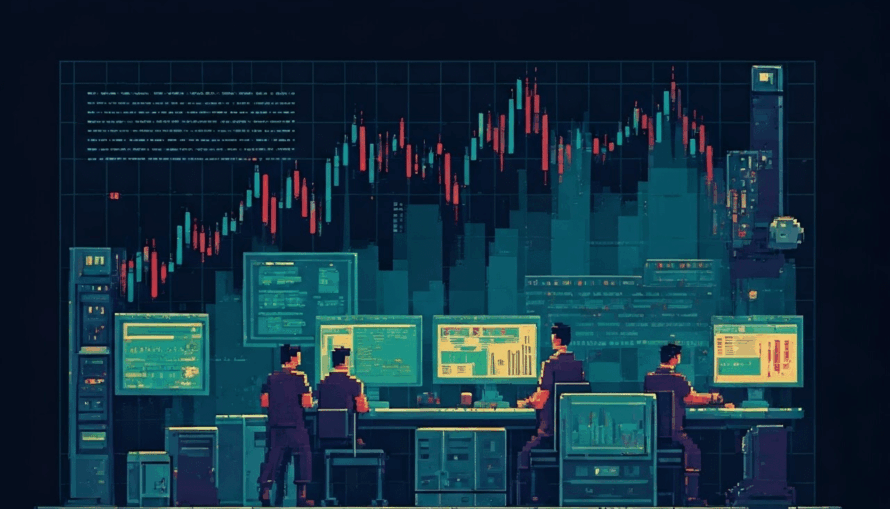
Key Highlights
Algo trading leverages algorithms to execute trades at high speeds, enhancing efficiency in financial markets. While it offers significant benefits such as reduced emotional trading and lower transaction costs, it also carries potential systemic risks and high initial costs. The success of algo trading relies heavily on robust software, data feeds, and a thorough understanding of trading algorithms. Future trends indicate an increasing integration of machine learning and blockchain technology in algo trading strategies. Understanding the regulatory environment is crucial for successful algo trading in India.
Introduction
Algorithmic trading has changed how people interact with financial markets. It uses complex math models and software to make trades much faster than before. As technology gets better, this method reduces human errors and improves the effectiveness of trade orders by using algorithms. Trading strategies keep evolving, and with artificial intelligence included, the world of algo trading stays lively. It adjusts to different market conditions and provides unique chances for both institutional and retail traders. To navigate today’s trading environment, it’s important to understand this new approach.
Understanding Algo Trading
Algorithmic trading, or algo trading, uses math and automated systems to make trades in financial markets. This method depends on set conditions to look at past data and market conditions. This helps in making trades at the right time. By using computer programs, algorithmic traders can take advantage of market volatility, reduce risks, and improve their trading styles. Different trading algorithms are created for various financial instruments. They help in managing trade orders effectively and lower human errors during the trading process. The use of technology has changed traditional methods, making trading strategies more advanced.
Definition of Algo Trading
Algorithmic trading means using computer programs and math models to carry out trade orders in financial markets. These algorithms look at a lot of historical data and current market conditions. They use predefined conditions and technical indicators to find the best times to enter and exit trades. This method allows for fast trading, which helps cut transaction costs and lower human errors. Algorithmic trading can be used in various markets, like stocks, options, and futures. It improves trading efficiency for both institutional and retail traders.
Brief History and Evolution of Algo Trading
The start of algo trading goes back to the 1970s. During this time, exchanges used automated systems to make trades work better. In the 1980s and 1990s, technology improved. This led to institutional investors, like pension funds and hedge funds, using algorithmic trading strategies. They relied on historical data and mathematical models for their trading decisions. In the 2000s, more computers became available. This change brought more people into the market and increased competition. Today, algo trading is a key part of the trading process. It uses complex algorithms to handle different market conditions easily.
How Algo Trading Works
Algorithms enhance trading by placing orders at optimal times, increasing potential profits and reducing transaction costs. They analyze historical data and market conditions to make swift decisions based on predefined rules, unlike human traders.
Each algorithmic trading strategy is tailored for specific markets and adapts to volatility changes. The combination of machine learning, data feeds, and fast execution allows algorithmic traders to capitalize on rapid opportunities in financial markets efficiently.
The Role of Algorithms in Trading
The use of algorithms in trading is changing how markets work. These smart computer programs look through large amounts of historical data. They find trading opportunities based on specific conditions. Algorithms help make better choices about trade orders like entry and exit points. They also help cut down on transaction costs and human errors. This results in better efficiency when carrying out strategies in various markets. Algorithmic traders can quickly respond to market volatility and changing situations. This has a big impact on the trading process.
Types of Algorithmic Trading Systems
Different algorithmic trading systems have unique roles in financial markets. Execution algorithms aim to improve trade orders based on set factors like price and volume. This helps to lower transaction costs. In contrast, market-making algorithms provide buy and sell quotes to ensure there is enough liquidity. They profit from the difference between buying and selling prices. Statistical arbitrage systems use mathematical models to find price differences in various securities. Meanwhile, trend-following algorithms take advantage of market momentum. Each type uses historical data and predefined conditions to make trading better and reduce human errors. This is key for today’s trading strategies.
Pros of Algo Trading
Efficiency is a big benefit of algorithmic trading. It lets orders be executed very quickly. This means it can react to market conditions much faster than a human trader. This quick execution often leads to lower transaction costs and more profit.
Another key advantage is that it helps reduce emotional trading decisions. Traders can use predefined conditions in trading algorithms. This helps them avoid making choices based on feelings. Instead, they can rely on mathematical models and historical data. This smart way of trading encourages discipline, which is very helpful in busy securities markets.
Efficiency and Speed
Using trading algorithms makes the trading process faster and more efficient. These algorithms can look at a lot of historical data and market conditions in real-time. They can execute trade orders almost instantly. This quick response to market volatility helps algorithmic traders take advantage of short-lived opportunities and reduce transaction costs from human delays. Unlike manual trading, automated systems can keep track of many securities all the time. This ensures that predefined conditions are met without human errors, improving performance in various markets.
Reducing Emotional Trading Decisions
Emotional decisions in trading can lead to poor results. Our feelings can affect our judgment, especially when the market changes. Using algorithmic trading systems can help traders avoid these emotions. They focus on set rules and mathematical models to carry out trade orders. This method helps algorithmic traders stick to their trading strategies. It reduces the risks that come from impulsive choices. As a result, the trading process becomes more structured and based on facts. This leads to better risk management and improves financial results over time, which is especially important in volatile market conditions.
Cons of Algo Trading
Algorithmic trading offers several benefits, but also poses downsides, notably systemic risk. Linked algorithms can exacerbate market volatility during downturns or unforeseen events. Additionally, the high initial costs of developing and maintaining advanced trading systems can be prohibitive for smaller retail traders. Effective risk management strategies are essential to mitigate the negative impacts of relying solely on automated trading.
Potential for Systemic Risk
Systemic risk in algorithmic trading comes from how financial markets are connected and how quickly trades are done. When many algorithms react to market conditions at once, it can cause big price changes. This can harm the overall stability of the market. A clear example of this was the Flash Crash of 2010. During that event, automated trading led to a sudden drop in stock prices. Also, using historical data can sometimes miss unexpected market volatility, making risk management harder. It’s important to have safety measures in place to reduce these systemic risks.
High Initial Costs
Setting up an algorithmic trading system often needs a lot of money at the start. You will need good computers, special algo trading software, and strong data feeds. You might also need to hire skilled people, like quantitative analysts or developers, to build and keep up with the complex trading algorithms. These costs can be too high for retail traders. This makes it hard for them to compete with institutional investors, who usually can handle these costs better. Additionally, high transaction costs and ongoing maintenance costs can make it hard to keep making money with algo trading.
Key Components of Algo Trading
Key parts are important for how well algo trading works. Strong algo trading software is essential; it helps traders use complex trading strategies easily. Good data feeds are also needed. They give trading algorithms access to real-time and historical data, which helps in making accurate predictions and improving performance. With the right technology in place, these factors work together. They make the trading process better, lower transaction costs, and allow quick trade orders based on predefined conditions.
Software and Platforms
Many software and platforms help with algorithmic trading. They allow traders to carry out their strategies effectively. These tools connect easily with data feeds. They give quick access to historical data and market conditions, which are important for making smart choices. More advanced algo trading platforms use machine learning and strong mathematical models to improve trading algorithms’ efficiency. Also, easy-to-use interfaces help both retail and institutional investors handle their trading tasks well. This lowers transaction costs and ensures trade orders fit predefined conditions. These improvements play a big role in changing financial markets.
Data Feeds and Technology Infrastructure
Algorithmic trading depends a lot on good data feeds and technology. High-quality, real-time data helps traders check market conditions and place trade orders when certain rules are met. It means using different data sources like price feeds, historical data, and technical indicators in their trading algorithms. A good algo trading platform improves how fast trades happen and lowers transaction costs. This lets algorithmic traders take advantage of market changes quickly. Using strong technology also helps make sure everything runs smoothly and reduces human errors in the trading process.
Popular Algo Trading Strategies
There are different strategies for algorithmic trading. Each one is designed to take advantage of specific market conditions. Trend following strategies focus on the price movements of securities, which help traders go along with existing trends. Arbitrage happens when there are price differences between different markets or securities. Traders can use this to make a profit. Statistical arbitrage uses mathematical models to predict good price movements. This is based on historical data and technical indicators. All of these methods help algorithmic traders improve their trading processes. They also help lower transaction costs and manage risk better.
Trend Following Strategies
Trend following strategies take advantage of the natural momentum in financial markets. This helps algorithmic traders make money from price movements in one direction. They use mathematical models and look at historical data to find trends based on set rules. Traders can place trade orders automatically if the price keeps moving in the way they expect. This reduces human errors and makes the process faster. This method also lowers transaction costs since trades are done quickly. This allows market participants like hedge funds and institutional investors to succeed in different market conditions.
Arbitrage Opportunities
Arbitrage opportunities happen when prices for the same financial instrument are different in various markets. Algorithmic trading takes advantage of these price differences. This allows traders to make profits before the prices even out. By using advanced algorithms, traders can automatically buy and sell, which helps catch profits that manual trading might miss. This method needs real-time data and quick transactions. This makes it perfect for the fast-moving financial markets and helps get the most returns on investment.
Statistical Arbitrage
Statistical arbitrage uses mathematical models and algorithms. These help find price differences among related financial instruments. Algorithmic traders look at historical data and market conditions. They then make many trade orders at the same time to benefit from temporary price mistakes. This strategy needs careful attention to average prices and predefined conditions for when to enter and exit trades. It also aims to reduce transaction costs while increasing profit possibilities. Because of this, statistical arbitrage is popular among institutional investors and hedge funds. This is especially true in fast-paced securities markets, where quick reactions to market volatility are necessary.
Regulatory Environment in India
In India, the Securities and Exchange Board of India (SEBI) controls the rules for algorithmic trading. SEBI requires strict compliance with these rules. Institutional investors, like hedge funds and mutual funds, need to register their trading algorithms. This ensures transparency and keeps the financial markets stable. The regulations aim to manage the risks of high-frequency trading while promoting stability in the market. Furthermore, retail traders using algo trading platforms must be careful. They need to follow predefined conditions to reduce human errors and avoid market manipulation.
Legal Aspects of Algo Trading
Automated systems and algorithms have a strict set of rules in financial markets. These rules help keep things fair and clear. Algorithmic traders must follow the guidelines provided by organizations like the exchange commission. This means they need to stick to the rules about trading practices and how they use trading algorithms. These algorithms should not take advantage of market flaws or change market conditions in a harmful way. Legal issues also require good risk management. Algorithmic trading strategies must fit with what market participants should do to keep the securities markets honest and strong.
Compliance and Ethical Considerations
Compliance and ethics are very important in responsible algo trading. Algorithmic trading requires following strict rules set by governing organizations like the exchange commission. Being open about trade orders and trading strategies is key to stopping market manipulation. This helps keep things fair for all market participants. There are also ethical issues regarding how automated trading might affect market volatility. Having a strong risk management plan and creating accountability can help address these problems. This will support sustainable trading practices in financial markets.
Challenges Facing Algo Traders
The world of algorithmic trading has its challenges. One big issue is that market conditions can change quickly. This means algorithms must be updated often. Another problem is overfitting. This happens when traders use too much historical data to create their trading algorithms. While this may make the algorithms perform better, it can cause issues when market dynamics shift. Because of this, algorithmic traders must manage risks well to protect themselves from problems in the securities markets.
Need for Continuous Optimization
The trading world is always changing. This means traders need to keep improving their algorithmic trading strategies. They look at historical data and market conditions to make their mathematical models better. This way, their strategies can match current trends. By changing their approach, traders can lower risks and improve their trade orders. This helps them do better in the fast-moving securities markets. When they regularly check their algorithms against set conditions, they can handle market changes better. This also helps cut transaction costs and boosts overall efficiency. Using machine learning makes it even better, as it allows for smarter and more flexible trading methods.
Risks of Overfitting
Overfitting is a big problem in algorithmic trading. It happens when mathematical models are too focused on historical data. When traders change their algorithms a lot based on how past trades performed, they can create a system that doesn’t work well in new market conditions. This can cause bad decisions when trading in real-time. As market volatility changes, an overfitted algorithm may struggle to manage risks or control capital. This can lead to missed chances or heavy losses. Understanding this risk is important for algorithmic traders who want to keep strong trading strategies.
Future Trends in Algo Trading
Advancements in machine learning and artificial intelligence are changing algorithmic trading. These technologies will lead to smarter trading algorithms. They can adjust to market conditions in real time. This helps in lowering transaction costs. Blockchain technology will also improve transparency and security in electronic trading. It will make trade orders and risk management better. As financial markets develop, algorithmic traders need to stay alert. They should change their strategies to use these advancements. At the same time, they must watch out for any risks that come with more automation.
Integration of Machine Learning and AI
The mix of machine learning and AI in algorithmic trading systems is changing financial markets. Smart algorithms use historical data to find patterns. They also automate trade decisions based on set rules. With natural language processing and predictive analytics, these systems can adjust to changes in market volatility right away. Machine learning helps with risk management by improving models to better predict price changes. This teamwork makes trading smoother, lowers transaction costs, and boosts overall performance for both institutional and retail traders.
Impact of Blockchain Technology
The rise of blockchain technology is set to change algorithmic trading. It will make financial transactions more transparent and secure. Blockchain works as a decentralized ledger. This helps reduce human errors and fraud, building trust among market participants. With smart contracts, trade settlements can happen automatically. This can lower transaction costs and make the trading process smoother.
This higher efficiency can help trading algorithms adapt to market changes faster. In the end, blockchain could change the future of algo trading. It will be beneficial for both institutional and retail traders.
Conclusion
In the fast-changing world of financial markets, algorithmic trading is an important step forward. It uses technology and data analysis to improve trading efficiency. By using trading algorithms, market participants can manage different market conditions more accurately. This helps cut down on human errors and biases. But there are challenges like potential risks to the whole system and the need for constant updates. As algo trading includes more machine learning and artificial intelligence, investors should pay attention to these changes. This way, they can change their strategies to take advantage of opportunities in this active trading environment.
Frequently Asked Questions
Can Beginners Start with Algo Trading?
Yes, beginners can begin with algo trading, but they need to understand trading strategies and algorithms well. Knowing programming languages and trading platforms is important. Taking courses or finding a mentor can help newcomers succeed in this complicated area.
What Skills Are Needed for Algo Trading?
Successful algo trading needs a combination of skills. Traders must be good at programming and math. They also need to know about trading strategies, data analysis, and risk management. Understanding algorithms and having experience with machine learning can really help in this technology-focused area.
How Does Algo Trading Affect Market Volatility?
Algorithmic trading can increase market volatility. This is because it executes trades very quickly and at high frequencies. When algorithms respond to market signals, they can cause big price changes. This is especially true during times of high volatility. These sudden price movements can impact both traders and long-term investors.